The powerful convergence of artificial intelligence (AI) and telecommunications is transforming how we connect, communicate, and access information. Whether it’s the ability to automate customer service, provide advanced analytics capabilities, or detect and prevent fraud, AI-driven telecom networks are unlocking new opportunities for businesses everywhere.
Artificial Intelligence (AI) In Telecommunications: Keys to Building a Telecommunication Infrastructure That Supports AI Share on X
But to truly leverage the potential of AI in telecommunications, companies must invest in building a modern telecom infrastructure that supports artificial intelligence. This means understanding the challenges and opportunities this technology poses and developing strategies for managing them.
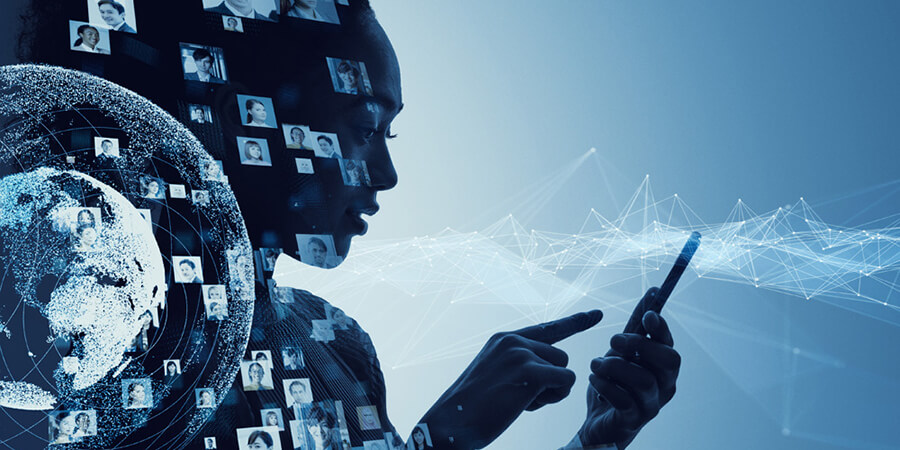
How Is AI Used in Telecommunications?
The telecommunications industry has always been at the forefront of technology innovation, and the emergence of AI has opened up an entirely new world of possibilities. Here are some of the ways AI is being used in telecommunications:
Automated Network Management
By analyzing data from network infrastructure, AI systems can take the guesswork out of network optimization, maximizing capacity and preventing outages. This leads to improved network performance and a seamless user experience.
With AI, telecommunications companies can ensure that their networks are running correctly by using predictive analytics to determine when certain equipment needs to be serviced or updated to guarantee better performance. In addition, companies can monitor how users access their network and see trending data to determine weak points that can be updated.
Intelligent Customer Service
AI-powered chatbots and voice bots are the future of customer service. Their ability to interpret customer inquiries and complaints means they can quickly provide resolutions without human intervention. This translates to faster response times, improved customer satisfaction, and cost savings for businesses in the telecommunications industry.
Monitoring and analyzing network traffic can be time-consuming and demanding for human operators. However, with the rise of IoT and edge computing, detecting and mitigating potential threats has become even more difficult. Fortunately, artificial intelligence can now automate this process and provide real-time network activity monitoring.
AI can flag suspicious behavior quickly and enable a faster response to potential security threats. By leveraging AI, businesses can improve network security and protect their assets effectively.
Improved Network Optimization
With the emergence of 5G and other new technologies, telecommunications companies must ensure their networks perform at their best. By utilizing artificial intelligence (AI), they can easily monitor customer usage patterns, device performance, and network activity.
This helps prevent network congestion, reduce latency, and ensure overall efficiency. With AI, telecom companies can optimize their networks to ensure that customers enjoy fast, reliable service, no matter how they choose to connect.
5G has the potential to support complex systems beyond traditional media platforms. Artificial intelligence (AI) plays a crucial role in optimizing this network. AI models can analyze device usage patterns and bandwidth consumption for each device connected to the network. By diverting bandwidth to frequently used applications, AI can optimize network performance and reduce latency.
Challenges With AI Infrastructure
As the use of artificial intelligence (AI) grows across industries, organizations face challenges in developing and maintaining an AI infrastructure that can support their needs. Below are some common challenges organizations face when implementing AI infrastructure.
Hardware vs. Virtual Servers
Organizations often face a dilemma when deciding whether to invest in expensive hardware or opt for more cost-effective virtual servers. While hardware offers better performance and storage, it can be costly and requires maintenance and upgrades.
On the other hand, virtual servers may be cheaper in the short term, but they may not match the performance levels of dedicated hardware.
Finding the right balance between cost and performance is essential for organizations seeking to maximize their resources. This decision should be based on the specific needs of the organization, its available resources, and long-term goals.
Centralized Training
Another challenge is coordinating centralized training for AI models. Centralized training requires large amounts of data to be transferred to a central location for processing, which can be time-consuming and resource-intensive. Organizations must also consider security concerns when transferring sensitive data.
Distributed Computing
Centralized training can be challenging for organizations, but distributed computing provides the answer. Organizations can significantly reduce the time and resources needed for training by processing data across several machines at once.
But implementing distributed computing requires specialized infrastructure and expertise that may not be readily available. Nevertheless, with careful planning and execution, organizations can achieve the desired performance from their AI models.
Hybrid Training
By using hybrid training, organizations can use centralized and distributed computing. This means they can process some data on local hardware while transferring other data to a distributed system, depending on their specific needs.
However, managing a hybrid infrastructure can be complex and challenging, requiring significant expertise. This can be a hurdle for organizations that lack the resources or personnel to manage such an infrastructure.
Modeling Techniques
Modeling techniques play an essential role in developing AI infrastructure. Below are three critical aspects of the modeling techniques that are essential for creating a telecommunication infrastructure that supports AI:
Extract, Transform, Load (ETL)
Building a successful AI infrastructure begins with data preparation. The Extract, Transform, Load (ETL) process is key to this preparation. ETL involves extracting data from multiple sources, then transforming it into a usable format before loading it into a data warehouse or analysis tool.
Why is ETL so important? First, it helps clean, consolidate, and standardize your data, making it easier to analyze and model. As a result, ETL plays a crucial role in data collection for customer behavior analysis, network performance analysis, and predictive maintenance analysis.
Centralized Initial Training
Centralized initial training is a powerful new approach to machine learning that lets you train models efficiently and cost-effectively. Training your models on a centralized server or cluster of servers can minimize the time and computational power required to get the job done.
And by optimizing your approach, you can ensure that your models are consistent, accurate, and up-to-date. By adopting centralized initial training, you can work more efficiently, reduce costs, and stay ahead of the game.
Pipeline Complexity and Maintenance Costs
When it comes to pipeline modeling techniques, complexity and maintenance costs are critical factors to consider. Models that are too complicated can be challenging to implement and maintain. As a result, companies can potentially face high costs and reduced efficiency.
However, telecommunication companies can overcome this challenge by developing models tailored to their specific needs rather than adopting generic models.
By streamlining their pipelines, these companies can reduce maintenance costs and improve overall efficiency.
Start Maximizing the Effectiveness of AI in Telecommunications
By leveraging the power of distributed computing, hybrid training, and ETL modeling techniques, telecom companies can maximize the effectiveness of AI in their operations. With proper planning and implementation, telecommunication companies can reap all the benefits that AI offers without sacrificing performance or increasing overhead expenses.
Recommended Read:
MUST-HAVE ONLINE TOOLS FOR DIGITAL MARKETERS IN 2023
HOW AND WHERE TO LEARN SEO IN 2023?
TOP 5 WAYS TO IMPROVE LOCAL SEO RANKING IN 2023
General FAQs
Artificial intelligence is being used to analyze data from network sensors to identify potential problems before they occur. This allows telecom providers to take proactive steps to fix problems and prevent outages.
Artificial intelligence provides a unique set of capabilities for infrastructure innovation, allowing for rapid and automated decision-making and improved network optimization.
Thanks to machine learning and deep learning, AI applications can learn from data and results in near real time, analyzing new information from many sources and adapting accordingly, with a level of accuracy that’s invaluable to business.